Logo
Undergraduate Study
- Who we AreWho we Are
- Research & Teaching Quality
- Our Strengths
- Our People
- Engagement & Place
- Faculties, Schools & Research Units
- Global
- Structure & Governance
- Locations
- Support for our Community
- Sustainability
- Facilities
- Equality, Diversity & Inclusion
- Social Justice
- Our Partnerships
- Newcastle Helix
- Art on Campus
- History of Newcastle University
- Education Strategy
- Study with UsStudy with Us
- Undergraduate
- Find a Degree
- Subject Areas
- Applications and Offers
- Step-by-Step Guide for UK Students
- Step-by-Step Guide for International and EU Students
- Applying through UCAS
- Entry Requirements
- Application Decisions
- Access Schemes and Pathway Programmes
- PARTNERS
- Policies and Procedures
- Applicants with Disabilities
- Mature Applicants
- Deferred Entry
- Undergraduate Application Advice
- Clearing
- Fees and Funding
- Scholarships and Bursaries
- Vice-Chancellor's Excellence Scholarships
- Vice-Chancellor’s EU Scholarships – Undergraduate
- Vice-Chancellor's Global Scholarships
- Vice-Chancellor's International Scholarships
- International Foundation Scholarships
- International Family Discounts
- Opportunity Scholarships
- Subject Scholarships
- Sports Scholarships
- St Nicholas’ Educational Trust Scholarship
- NU Sanctuary Scholarships
- Tuition Fees and Costs
- Student Loans
- International Student Finance
- Scholarships and Bursaries
- Undergraduate Open Days
- Sign up and Discover
- School and College Outreach
- Information for Parents and Supporters
- Request a Prospectus
- Postgraduate
- Global Scholarships
- Doctoral College
- Distance Learning
- Continuing Professional Development (CPD)
- Our Campus
- Student Life
- Student Blog - Belong
- Accommodation
- Your Future
- Work Placements
- Careers Service
- About the Careers Service
- Careers Service Events
- Work for Yourself
- Career Planning
- Careers Modules
- Making Applications
- Interviews, Tests & Assessment Centres
- Internships, Placements & Shadowing
- Finding Jobs
- Handling Job Offers
- Researching Employers
- Making Contacts
- Further Study
- Awards, Competitions & Project Funding
- Volunteering
- Boost Your CV
- Defence Technical Undergraduate Scheme (DTUS)
- Meet Us
- International Students
- International Pathway Courses
- Student Exchange and Study Abroad
- Request a Prospectus
- Key Dates
- Contact Us
- Your Academic Experience
- Undergraduate
- Our ResearchOur Research
- Research Impact
- Research Strengths
- Centres of Research Excellence
- Centre for Ageing and Inequalities
- Centre for Biomedical Engineering
- Centre for Cancer
- Centre for Children and Youth
- Centre for Climate and Environmental Resilience
- Centre for Cyber Security and Resilience
- Centre for Data
- Centre for Energy
- Centre for Healthier Lives
- Centre for Heritage
- Centre for Landscape
- Centre for Mobility and Transport
- Centre for Rare Disease
- Centre for Researching Cities
- Centre for Transformative Neuroscience
- Centre for Water
- Research Culture
- Doctoral College
- Global Challenges Academy
- Research Excellence Framework (REF) 2021
- Research Directory
- Research Governance
- Research and Innovation Support
- Research Facilities
- Research Funding
- Research News
- Contact Us
- Work with Us
- Alumni
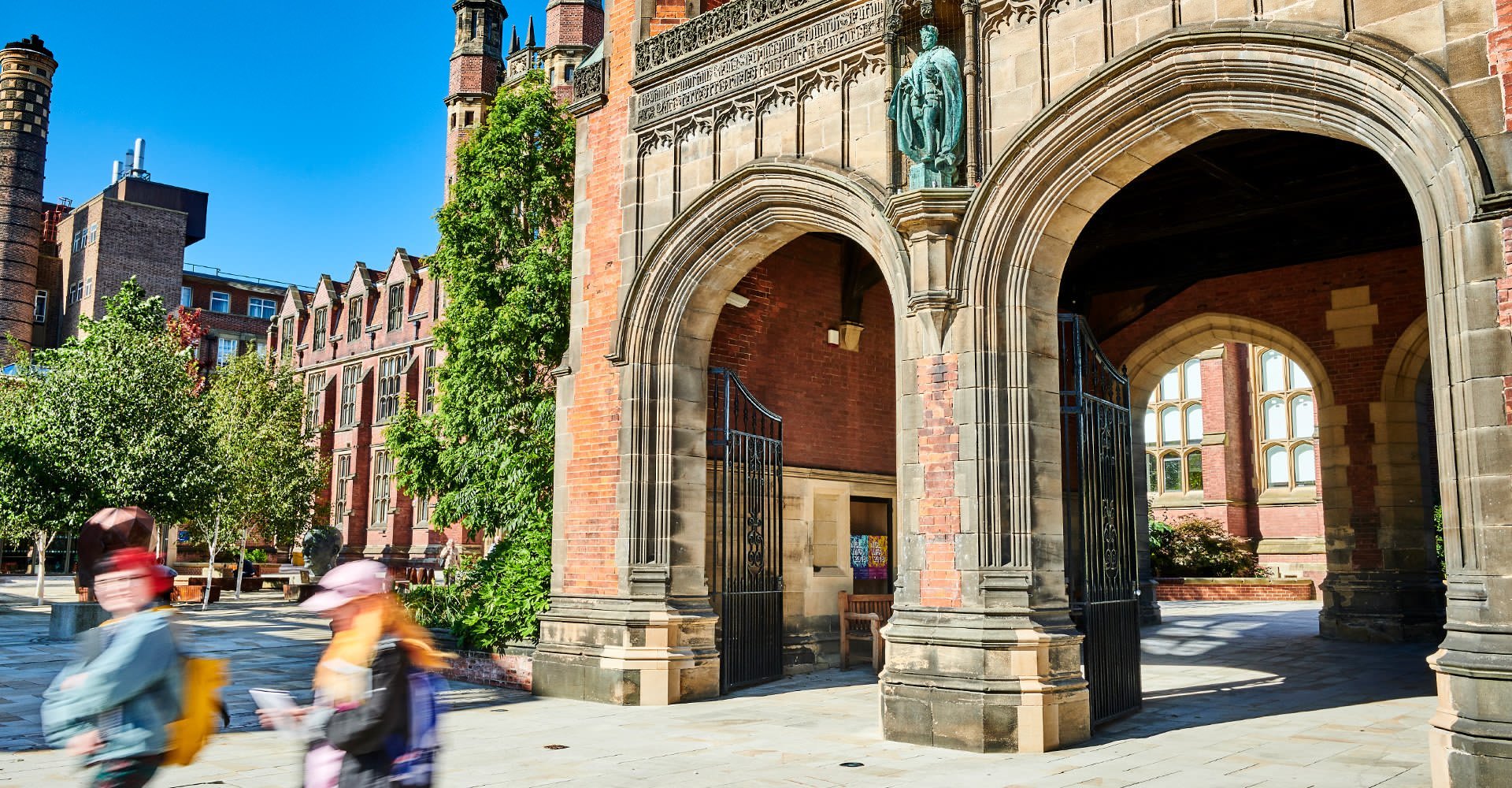
No modules have been found with the code: MAS3913
Error:404
- Please visit the Module Catalogue to search our full selection