- Who we AreWho we Are
- Research & Teaching Quality
- Our Strengths
- Our People
- Engagement & Place
- Faculties, Schools & Research Units
- Global
- Structure & Governance
- Locations
- Support for our Community
- Sustainability
- Facilities
- Equality, Diversity & Inclusion
- Social Justice
- Our Partnerships
- Newcastle Helix
- Art on Campus
- History of Newcastle University
- Study with UsStudy with Us
- Undergraduate
- Find a Degree
- Subject Areas
- Applications & Offers
- Step-by-Step Guide for UK Students
- Step-by-Step Guide for International & EU Students
- Applying through UCAS
- Entry Requirements
- Application Decisions
- Access Schemes & Pathway Programmes
- PARTNERS
- Policies & Procedures
- Applicants with Disabilities
- Mature Applicants
- Deferred Entry
- Undergraduate Application Advice
- Clearing
- Fees & Funding
- Scholarships & Bursaries
- Subject Scholarships
- Sports Scholarships
- Opportunity Scholarships
- VC's Excellence Scholarships
- VC's Global Scholarships
- VC's International Scholarships
- International Foundation Scholarships
- St Nicholas’ Educational Trust Scholarship
- NU Sanctuary Scholarships
- Undergraduate Norway Scholarship
- International Family Discounts
- VC’s EU Scholarships – Undergraduate
- VC's Excellence Scholarships - Europe
- VC's Business Excellence Scholarships - Europe
- Cowrie Foundation Scholarship
- Edward Long Scholarship
- Tuition Fees & Costs
- Student Loans
- International Student Finance
- Scholarships & Bursaries
- Sign up & Discover
- School and College Outreach
- Information for Parents and Supporters
- Postgraduate
- Doctoral College
- Distance Learning
- Continuing Professional Development (CPD)
- Our Campus
- Student Life
- Student Blog - Belong
- Accommodation
- Your Future
- Work Placements
- Careers Service
- About the Careers Service
- Careers Service News
- Careers Service Events
- Work for Yourself
- Career Planning
- Careers Modules
- Making Applications
- Interviews, Tests & Assessment Centres
- Internships, Placements & Shadowing
- Finding Jobs
- Handling Job Offers
- Researching Employers
- Making Contacts
- Further Study
- Awards, Competitions & Project Funding
- Volunteering
- Boost Your CV
- Defence Technical Undergraduate Scheme (DTUS)
- Meet Us
- International Students
- International Pathway Courses
- Student Exchange and Study Abroad
- Request a Prospectus
- Key Dates
- Contact Us
- Your Academic Experience
- Undergraduate
- Our Research
- Work with UsWork with Us
- Case Studies
- Expert Solutions
- Procuring Goods & Services
- Business and Partnerships
- Health & Social Challenges
- Creative Collaborations
- Alumni
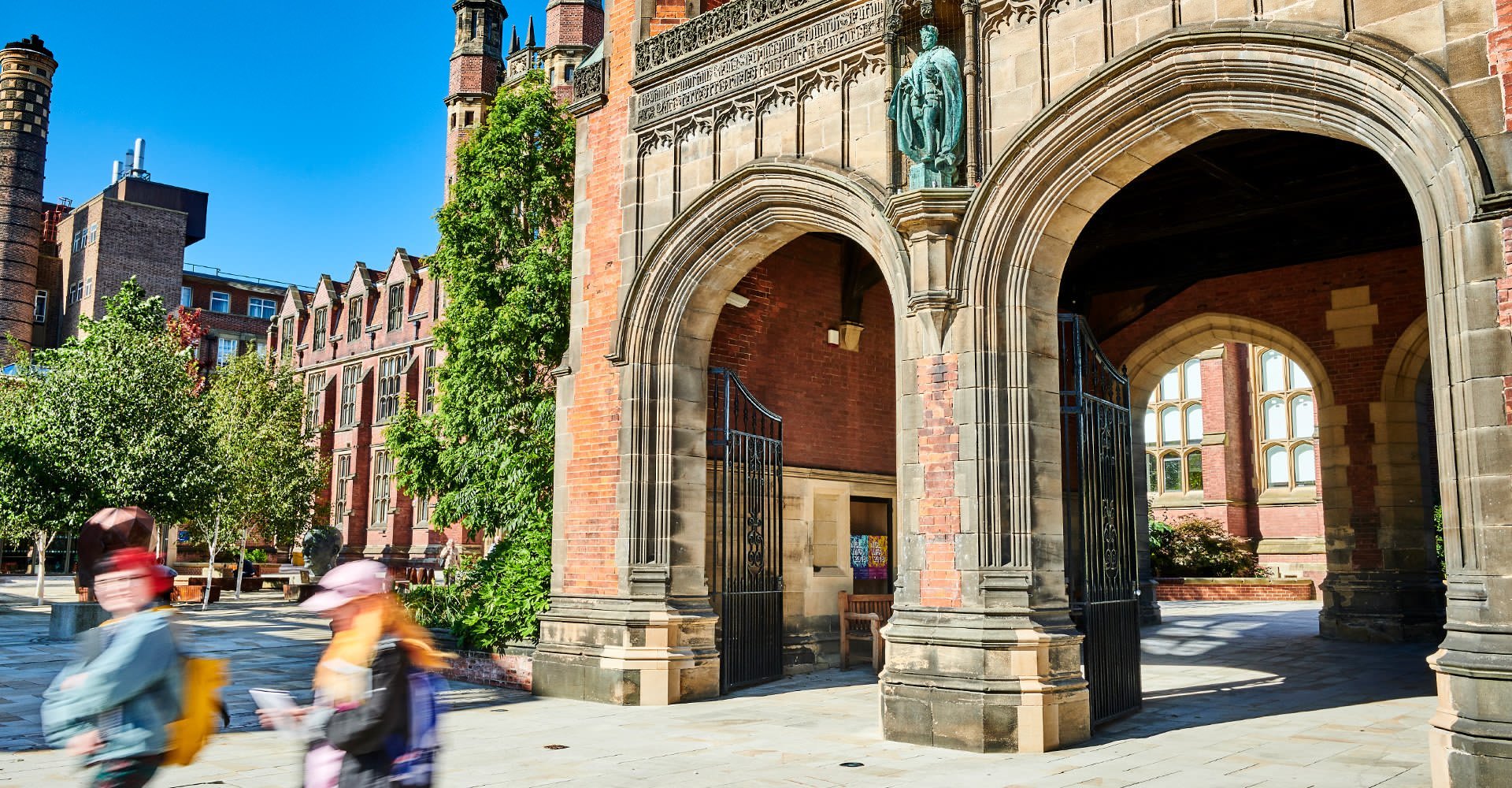
No modules have been found with the code: MAS8910
Error:404
- Please visit the Module Catalogue to search our full selection